The Memo - Special Edition - ARK AI report - 11/Mar/2023
ARK Invest: Big Ideas 2023! Annotated by Alan
FOR IMMEDIATE RELEASE: 11/Mar/2023
Welcome back to The Memo.
Let’s run through ARK’s latest report featuring a big section on AI.
Title: ARK Invest: Big Ideas 2023!
Chapter: Artificial Intelligence: Creating The Assembly Line For Knowledge Workers
By: Will Summerlin, Frank Downing
Date: 31/Jan/2023
URI: https://ark-invest.com/big-ideas-2023/artificial-intelligence/
Alan: ARK Invest (wiki) is no stranger to controversy, with owner and founder Cathie Wood overseeing a 70% drop in the value of funds under management in 2021-2022. However, their written reports are excellent, and I’ve found them to be both accessible and rigorously researched.
Here are some of my comments on the AI section, pages 20-29.
Alan: DALL-E 2, Meta MAV, and SD 2.0. No single researcher can cover everything (I’m finding that out the hard way!), but this is a very limited view of Generative AI in 2022. There were more impressive models than the three above, and there were also far more of them.
150,000 transformer models were made available in 2022 according to Hugging Face.
Additionally, there were more than 130 new papers published every day on arXiv.org.

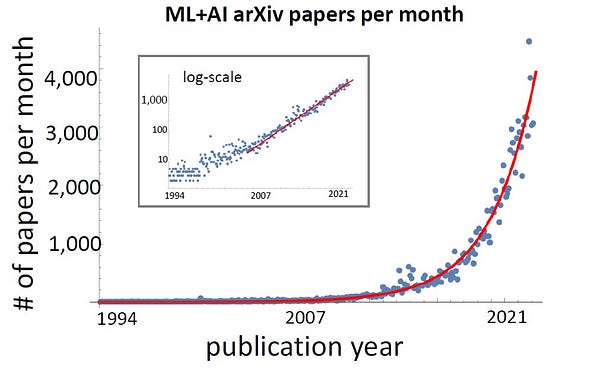
Alan: This is an excellent quantification of costs for graphic design; from $150 and 5 hours (human) to 8c and 1 minute (AI). These kinds of comparisons are necessary for people everywhere, but I’ve found them to be particularly valuable for highlighting massive changes when presenting to government ministers, executives, and other decision makers.
Alan: Note the GPT-3 training costs drop from $4.6M to $0.4M within 24 months, and the forecast of $30 by 2030. Obviously, we want to continue to outperform GPT-3, but the quantification (and visualization) is useful to see.
Alan: No further comment, except to note that both Google TPUv4 and NVIDIA Hopper H100 circuits were designed and optimized by AI (see my AGI page). This contributes to both lower hardware costs and lower compute costs.
Alan: This is a poor slide. It says:
GPT-3 had 175B parameters and 300B tokens. It cost $4.6M to train.
The next model may have 10,000B (10T) parameters and 216,000B (216T) tokens. The source is Chinchilla (my explanation). It may cost $0.6M to train.
DeepMind’s MassiveText (multilingual) is now 5T tokens, estimate 22.3TB, and would still be the largest text dataset in the world as of Q1 2023.
To get to 216T tokens, we would need to source just under 1 petabyte (963TB or 963,000GB) of filtered data. This is made possible when bringing in transcribed video (audio) from places like YouTube and film libraries via OpenAI Whisper (paper) or Google’s new USM announced in Mar/2023 (paper). Additionally, models like Gato flatten multimodal tokens (like button presses or robot arm movements) into text.
Alan: Data has always been a big focus for training post-2020 AI models. The big players have access to unusual and significant amounts of data measured in petabytes (thousands of terabytes or millions of gigabytes): Google and DeepMind (via Alphabet), OpenAI and Microsoft, and even Meta AI.
Alan: I use some of these metrics in some keynotes for developers. The full source is available on the GitHub Blog (7/Sep/2022). I believe that the 10x multiplier on developer productivity—to 2030 according to ARK—will actually be completed in the next few months (e.g. 2025-2026). See also ChatGPT self-improving code in Microsoft’s embodiment experiments (20/Feb/2023), as well as my conservative countdown to AGI.
Alan: Note that all of ARK’s forecast time scales seem out-of-whack. 2030 is a very round number, and perhaps it will take that long for ‘mass adoption’ and full acceptance into society (however that is measured). The actual arrival time for this is again measured in months from now (e.g. 2025-2026); and some of it is already either in place or possible with optimizations like the ones OpenAI is implementing for ChatGPT (e.g. now less than a fifth of a cent per token).
Alan: They’ve hidden the lede here. In the opening paragraph, ARK says:
At 100% adoption, AI spend of ~$41 trillion could increase labor productivity ~$200 trillion, dwarfing the ~$32 trillion in knowledge worker salaries and rivaling current projections of global GDP in 2030. If vendors were to capture 10% of value created by their products, AI software could generate up to $14 trillion in revenue and $90 trillion in enterprise value in 2030.
All my very best,
Alan
LifeArchitect.ai
Archives | Unsubscribe new account | Unsubscribe old account (before Aug/2022)